Bad Stats are Holding Back Web 2.0
I use “stats” generically here because, at its simplest meaning, what is holding back web 2.0 is bad statistical planning. You have to ask the right questions with the right controls to get the right answers so you can make the right recommendations. The napkin business plan generation apparently has not learned its lesson in the web 2.0 sphere, with new sites and communities launching by the dozens. Unfortunately, web 2.0 communities commonly make the following assumption: massive data, regardless of its quality, is sufficient.
What we need is a more complete measurements (questions) and common, controlled subjects (wines).
To illustrate this point, I would like to identify 3 wine Web 2.0 communities (Corkd – BottleNotes – TasteVine), 2 of which do it the “wrong way” and one of which does it the “right way”. To my knowledge, no other industry or web 2.0 site is using the same methodology as the 3rd site, and it will make all the difference in the world. Full Disclosure: Virante worked on the development of TasteVine, so, while I believe my statements to be accurate and worth looking at, you should investigate them yourself.
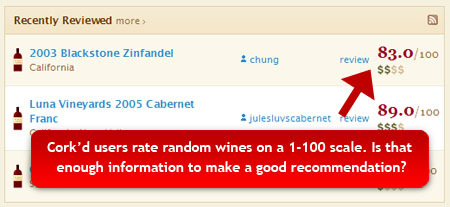
1. Corkd.com: (the Netflix model)
This is the most common form of web 2.0 data gathering and recommendation generating that occurs on the web. It works on this logic…
- Get a ton of people to rank stuff 1-100 (formerly 1-5)
- Find people with similar rankings
- Create recommendations based on that data
The Netflix model presents the obvious long-term consequences: needing to run a $1,000,000 prize to get better recommendations from their data. The problem is not bad algorithms, it’s bad data.
In the case of wines, one individual may like sweet wines and give it a 100 while another likes dry wines and give it a 100. It would take massive numbers of duplicate tastings and rankings to build a decent recommendation based on these poor measurements.
The NetFlix/Corkd model compares apples to apples, but one person is looking at smell, another at taste, and yet another at color. Unless you can tease out WHY a person feels one way or another, you can’t use their opinions to make recommendations for others.
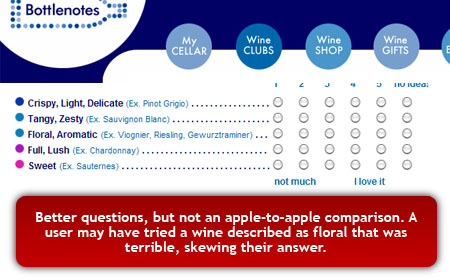
2. Bottlenotes : (The EHarmony Model)
This is a growing model of match-making methods but presents a similar set of problems. The gist is simple: give everyone a test at the beginning and join people together.
For the sake of argument, lets say you ask a person whether or not they like sweet wines as part of the profiling system. They say yes, but their tastes are actually skewed greatly because they consider only the very sweetest of wines to be sweet.
With the EHarmony model, the problem is you are not comparing apples-to-apples. You are asking questions about the individual’s subjective reality, with no fixed subject which we can all agree upon. Do you like tart, do you like sweet, do you like crisp, do you like ripe? This measurements are more complete, but the subjects vary.

3. TasteVine: The, uhhh, TasteVine model.
Imagine how good E-Harmony would be if everyone who signed up dated one of 10 people for a day and then rated them. Instead of obtuse, ambiguous questions about values, we would have real world tests. Unfortunately, this just isn’t possible in the realm of online dating. However, for wines, this is definitely a possibility.
The TasteVine model fixes the majority of these basic statistical problems from the onset…
- Taste the exact same wines everyone else does (1 from each varietal)
- Asks the same questions about the wine (is it sweet, is it smooth, do you like it)
- Create a TasteID based on this data to match with other users (your TasteBudds)
- Deliver recommendations based on reviews of other wines by your TasteBudds
It does require that 1 extra step, that you try some of the 12 wines to get better recommendations. However, this is correct method to gather and use data.
It will be interesting to see how this community fairs in the long run. It definitely has the best recommendation algorithm, but the user does have to taste a few wines to get that value out of it. However, even in competitor sites like Cork’d, the user would have to try a large number of wines to start building up an accurate profile from which the system can make recommendations. We shall see.
No tags for this post.5 Comments
Trackbacks/Pingbacks
- Heat Wave Roundup | Power Webblog - [...] this post from Russ Jones - Bad Stats are Holding Back Web 2.0. His example of the one Web…
I fully agree with your ideas here. Without having a common ground to rate information then there really is no purpose. On a very similar level, there is a lot of ambiguity in local search and review based websites because more people will go out to rate/review something after a bad experience than they will a good experience. Overall this skews the rating system and the results because everything is dragged downward many times.
Sounds interesting. I’m not sure if TasteVine does it, but it would be a neat idea to have a way to purchase the 12 wines right when you join, maybe at a discount if it’s possible. Not required, but it still would be a neat option.
I think the Netflix model works great for Netflix, because it’s so easy to watch zillions of movies.. Whereas wine it’s not so easy to drink zillions of wines, unless you lived next to a winery or have tons of cash =P
Note that the Cork’d rating also corresponds to Wine Spectator’s point system that is used on and off line and familiar to consumers.
I like the NetFlix model – you have to drink lots of wine! 😉
I do like the idea of a starter kit though – you could even have several to choose from. If you like old world reds, australian shirazs or only want bottles under $10 you should be able to pick the appropriate starting point and be matched against others with choosing the same option.
I wonder how much the audience has been factored into the stats here though? Are people likely to use it to try/explore new wines, or do people get a kick out of disagreeing with other people? I suspect a number of people are likely to keep it as their own personal drinking record so they can remember which wines they liked and which they didn’t, in which case the relationship to others is secondary.
My .02 cents:
I feel your pain regarding bad stats, its difficult to make something from nothing… Also, regarding the fixed sample of 12 wines, I think that is a good point for any recommender system. It seems like Netflix does something like that, since in the prize dataset there is a subset of movies almost everyone has rated (I think they show you a bunch of movies and ask you to rate 20 of them when you sign up).
That said, I think there are a few considerations you are missing here… There is a tradeoff between getting the most information possible and having a rating system people will actually bother to use. The Netflix/Amazon is an approach based on a lot of usability testing and is designed to get the most user feedback. The reality is that most people won’t take the time to fill out a number of ratings for each product. That said, if you find a way to get more data out of people, go for it.
You mention “In the case of wines, one individual may like sweet wines and give it a 100 while another likes dry wines and give it a 100. It would take massive numbers of duplicate tastings and rankings to build a decent recommendation based on these poor measurements.”
You’d be surprised at what you can do given simple 1-5 ratings…and you don’t need as many as you think (although the more data the better). Browse through the Netflix Prize forum to get some examples of how these different features can be extracted from ratings along just one dimension. Some users have as few as 4 or 5 movie ratings and their preferences can be predicted fairly well.
Again, the reason they can do this is because they have a lot of data, which was obtained by using a simple rating method. If they used a more complicated form, the response rate would most likely tank. Also, look at some of the work Google is doing in this area… in general they find that more data trumps a better algorithm almost every time.